Intelligent pipelines: a harmony of AI and human analytics
We are all experiencing a period of rapid technological advancement.
If you’ve kept an eye on the news recently, you would have seen that AI is a big part of this. When considering AI, it is important to remember that it is just one method of analysis that may not always be the best option. In this article, we’re exploring the different approaches pipeline operators can take when considering AI-driven asset optimisation.
We published an article on this in the October 2023 edition of World Pipelines Magazine – “Green and Lean: Data Driven Asset Optimisation for Transmission Pipelines”. The following is an edited extract, and you can get your hands on the entire article at the end.
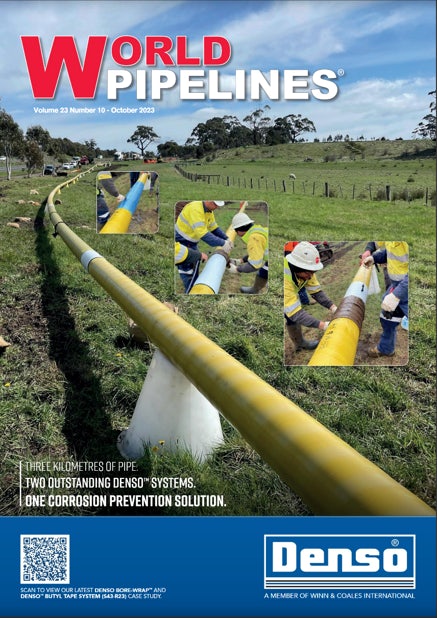
World Pipelines, October 2023
Artificial intelligence in pipelines
Rising energy prices induce increased operational costs. The energy industry faces immense pressure to lower OPEX and participate in global efforts to decarbonise. Pipeline operations, the backbone of the global energy landscape, are no exception. With the rise of large-language models like ChatGPT, artificial intelligence (AI) is the tool on everyone’s minds. Embracing big data analytics and AI offers exciting opportunities for pipeline operators to unlock hidden insights for greater efficiency and reduced emissions.
AI relies on and benefits from large datasets for pattern recognition and predictive modelling. Pipeline operators already harvest vast amounts of data, such as flow and pressure, so they must exploit this resource. The use of big data analytics, in combination with AI algorithms, allows operators to harness the power of their available data for a more comprehensive understanding of pipeline behaviour and optimised operations.
Three AI-driven techniques
In the world of AI-driven asset optimisation, pipeline operators face a choice between three approaches:
- Adopting classical analytical methods,
- Pure artificial intelligence (AI) models,
- A hybrid approach.
Evaluating the different AI techniques
The more traditional analytical methods can be alluring thanks to their relatively straightforward implementation and predictable, deterministic outputs. They are also more reliable, especially when applied to older assets without historical data for AI training. However, these models struggle to account for the complexity and nuance of real-world situations, a weakness not shared by AI trained on real-world data.
AI methods are also more flexible regarding pipeline instrumentation, allowing the integration of far more data sources. AI methods can even compensate for some lacking inputs a classical model might insist upon. AI’s ‘black box’ nature, however, typically necessitates subsequent investigation rather than immediate action and results. Despite this, AI is an obvious choice for complex analysis challenges (such as predictive maintenance). The small sacrifices to informational reliability and repeatability are tolerable given the prohibitive costs to manually evaluate assets.
In many cases, a hybrid approach unites the best of both approaches.
Applications of AI for pipeline operators
There are many ways for operators to harness the power of AI and data analytics. One area that Klarian believes deserves particular attention is the optimisation of pipeline pumps and compressors. These unsung heroes play a pivotal role in the industry, and we believe it is high time we explore how big data analytics can transform their efficiency and sustainability for the better.
That’s why we offer a pump and compressor optimisation solution as part of our Digipipe service.
If you’re interested in reading the entirety of our article as featured in World Pipelines, sign up below and we’ll send you your own copy directly into your inbox.