Monitoring and Anomaly Detection Approaches with AI and Data Analytics for Pipelines
Klarian | Pipeline Technology Journal - 27 November 2023
Effective monitoring and anomaly detection are fundamental prerequisites for safeguarding the efficiency, integrity, and reliability of pipeline systems.
There’s a wide spectrum of approaches you can take for this. At one end, cutting-edge machine learning and, at the other, purely physics-based approaches. Curious about how best to strike a balance between the two? We’ve published a paper investigating exactly that in November 2023’s edition of the Pipeline Technology Journal (PTJ):
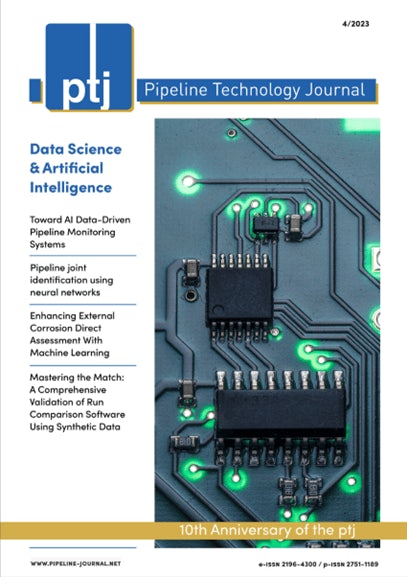
Article abstract
In the paper, we explore both physics-based and machine-learned approaches for operational asset monitoring and anomaly detection as well as evaluating their performance and appropriateness across a selection of analytical challenges. Specifically, we look at identifying and quantifying anomalies in pumping performance and orifice plate accuracy in relation to work completed for British Pipeline Agency (BPA) and a prominent UK gas operator.
Anomaly and outlier detection play a pivotal role in identifying abnormal events and observations that deviate significantly from expectations. It is crucial for identifying unusual occurrences and potential operational threats or malfunctions. Without effective monitoring, operators risk missing the important clues which could lead to environmental disaster and economic damage to loss of regulatory compliance and threat to human safety. This underscores the need for comprehensive research and technological advancements in this domain.
The physics-based models we investigated for BPA include computing hydraulic efficiency expectations versus reality, with cross-correlation of pumping and valve statuses, and breaking complex networks into legs and routes for a granular analysis, combined with a fuel-tracking system. For the gas operator, machine-learning techniques included multivariate statistical analysis using Principal Component Analysis (PCA) and the Mahalonobis distance, artificial neural networks (specifically autoencoder networks), and Support Vector Machines.
While each method has its own trade-offs, research proved that both physics-based and machine-learned methods are effective monitoring and anomaly detection approaches. Deployment is highly contextual, dependent on specific operational requirements, system complexity, and available data sources.